D Data-Driven UX: Optimizing Decisions Through Data Analysis
Por Redacción Aguayo
Explore the Fundamental Laws of UX that every designer should know to enhance user experience. From the Hick's Law to the Miller's Law, learn how to apply these principles in your design.
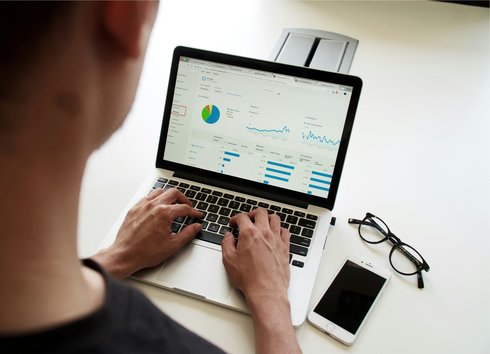
Introduction to Data-Driven UX
User Experience (UX) has evolved considerably in recent decades, transitioning from a discipline focused on intuition and creativity to one based on data. Data-driven UX refers to the practice of using quantitative and qualitative information collected from user behavior and interactions to make informed decisions about the design and optimization of digital products and services.
The Evolution of UX
UX used to be primarily based on intuition and the designer's experience, often leading to subjective decisions. However, with the advent of digital technology and massive data-driven collection, UX professionals have adopted a more evidence-based approach to understand and improve user experience. This has led to the emergence of data-driven UX as a more robust and effective approach to digital product design.
Defining Data-Driven UX
Data-driven UX focuses on using data to better understand how users interact with a digital product or service. This involves collecting data on user behavior, such as clicks, actions, time spent on a page, conversions, and more. Additionally, it also involves collecting qualitative data through surveys, interviews, and user testing to understand user perceptions, attitudes, and needs.
Benefits of Data-Driven UX
One of the main benefits of data-driven UX is its ability to make evidence-backed decisions. By collecting and analyzing data on user behavior and preferences, designers can identify areas for improvement and make informed decisions to optimize user experience. This can lead to more effective and satisfying products and services for end users.
The Role of Data in the Design Lifecycle
Data plays a crucial role in every stage of the design lifecycle. From initial research to iteration and continuous optimization, data informs and validates design decisions. By understanding how users interact with a product or service at each stage, designers can identify opportunities for improvement and optimization to ensure an exceptional user experience.
Data-driven UX represents a powerful and effective approach to the design of digital products and services. By leveraging quantitative and qualitative data, designers can better understand user needs and preferences, leading to more effective and satisfying products. In the following chapters, we will explore in detail the tools, techniques, and best practices associated with data-driven UX.
Importance of Data Analysis in User Experience
Data-driven analysis plays a fundamental role in improving and optimizing the user experience (UX) in digital products and services. As the digital world becomes increasingly complex and competitive, the ability to understand and adapt to user needs and expectations has become crucial for the success of any online platform or business. In this chapter, we will explore the importance of data-driven analysis in UX and how it can positively impact design and user satisfaction.
Understanding User Behavior
One of the most important aspects of data-driven analysis in UX is its ability to provide detailed insights into how users interact with a digital product or service. By tracking key metrics such as time spent on page, actions taken, navigation patterns, and conversions, designers can gain deep insights into user behavior. This understanding allows them to identify areas for improvement and make informed decisions to optimize the user experience.
Personalization and Adaptation
Another crucial aspect of data-driven analysis in UX is its ability to facilitate personalization and adaptation of content and functionality based on individual user needs. By collecting and analyzing data on user preferences and behavior, companies can deliver more relevant and personalized experiences. This can range from product recommendations to adjustments in the user interface to better fit each user's preferences.
Identifying Pain Points
Data-driven analysis is also essential for identifying and addressing pain points in the user experience. By analyzing behavioral data, designers can detect areas of the product or service that cause confusion, frustration, or difficulties for users. This may include usability issues, complex checkout processes, or unclear messaging. By addressing these pain points, companies can improve the user experience and increase customer retention and satisfaction.
Continuous Optimization
UX is a continuous and ever-evolving process, and data-driven analysis plays a key role in the ongoing optimization of digital products and services. By regularly monitoring and analyzing behavioral data, companies can identify emerging trends, assess the impact of updates, and make real-time adjustments to improve the user experience. This allows companies to stay agile and quickly adapt to changing user needs and expectations.
Conclusion
In summary, data-driven analysis is essential for understanding, improving, and optimizing the user experience in digital products and services. It provides valuable insights into user behavior and preferences, enables personalization and adaptation, identifies pain points, and facilitates continuous optimization. By integrating data-driven analysis into the design and development process, companies can create more effective, satisfying, and user-centered digital experiences.
Tools and Techniques for Collecting UX Data
Collecting user experience (UX) data is crucial to understanding how users interact with a digital product or service. There are various tools and techniques available that allow designers and analysts to collect data effectively and gain valuable insights into user behavior and preferences. In this chapter, we will explore some of these tools and techniques, highlighting their features, advantages, and how they can be used in different contexts.
User Behavior Analysis
One of the most common techniques for collecting UX data is user behavior analysis. These records, which can include data such as clicks, mouse movements, interaction times, and conversions, provide a detailed view of user behavior while navigating a website or using an application. Tools like Google Analytics, Hotjar, and Crazy Egg allow UX teams to analyze these records and gain insights into how users interact with the product.
Surveys and Questionnaires
Another popular technique for collecting UX data is through surveys and questionnaires. These tools allow designers to gather direct feedback from users about their needs, preferences, and experiences. Surveys can be sent via email, integrated into a website or application, or distributed through social media. Tools like SurveyMonkey, Typeform, and Google Forms make it easy to create and distribute surveys, as well as analyze the collected data.
User Interviews
User interviews are a qualitative technique that allows designers to obtain detailed information about users' needs, motivations, and expectations. During an interview, users can provide contextual and insightful information that can be invaluable for understanding their experience with a product or service. Interviews can be conducted in person, over the phone, or via video conference, and can be structured or unstructured. Tools like Zoom, Skype, and Google Meet facilitate the remote conduct of user interviews.
Usability Testing
Usability testing is a fundamental technique for evaluating user experience and identifying areas for improvement in a digital product or service. During a usability test, participants perform specific tasks while an observer records their actions and collects feedback. These tests can be conducted in specialized labs or remotely through software tools. Some popular tools for usability testing include UserTesting, UsabilityHub, and Optimal Workshop.
Social Media Analysis and User Feedback
Social media analysis and user feedback are important techniques for collecting real-time UX data and understanding how users perceive a product or service. Social media platforms like Twitter, Facebook, and Instagram provide a wealth of data about user conversations and opinions. Additionally, comments and reviews on websites and app stores can offer valuable insights into user satisfaction and pain points. Social monitoring tools like Hootsuite, Sprout Social, and Brandwatch facilitate the tracking and analysis of this data.
In conclusion, collecting UX data is essential for understanding and improving the user experience in digital products and services. The tools and techniques mentioned above provide designers and analysts with the necessary tools to collect data effectively and gain valuable insights into user behavior and preferences. By using these tools and techniques intelligently and strategically, UX teams can make informed, user-centric decisions that enhance the overall product experience.
Quantitative Analysis vs. Qualitative Analysis in UX
In the field of User Experience (UX), there are two main approaches to collecting and analyzing data: quantitative analysis and qualitative analysis. Both approaches are essential for fully understanding user behavior and needs, but they differ in their methods and objectives.
Quantitative Analysis
Quantitative analysis focuses on the collection and analysis of numerical and statistical data. This approach provides insights into the behavior of a large number of users, allowing for the identification of patterns, trends, and key metrics. Some common techniques of quantitative analysis include click tracking, web analytics, A/B testing, and conversion analysis.
Advantages of Quantitative Analysis:
- Scalability: It allows for the analysis of large amounts of user behavior efficiently.
- Objectivity: Quantitative data is objective and easily measurable, facilitating evidence-based decision-making.
- Pattern Identification: Helps identify patterns and trends through metrics and statistics.
Challenges of Quantitative Analysis:
- Lack of Context: It may lack context about why users behave in a certain way.
- Limitations in Depth: Often does not provide detailed information about user motivations and needs.
- Potential Biases: Quantitative data may obscure certain aspects of user behavior due to biases in metric design or data interpretation.
Qualitative Analysis
Qualitative analysis focuses on gaining in-depth insights into users' experiences, perceptions, and emotions. This approach uses techniques such as interviews, focus groups, usability testing, and content analysis to obtain detailed and contextualized information about user needs and desires.
Advantages of Qualitative Analysis:
- Depth of Understanding: Allows for a detailed and contextualized understanding of user needs and desires.
- Rich Context: Provides information about the context and motivations behind user behavior.
- Identification of Complex Issues: Helps identify complex issues that may not be evident in quantitative data.
Challenges of Qualitative Analysis:
- Resource-Intensive: Requires more time and resources than quantitative analysis due to its focus on depth and context.
- Subjectivity: Interpretation of qualitative data can be subjective and influenced by researcher biases.
- Limited Generalization: Qualitative findings may not be generalizable to the entire user population due to their exploratory nature.
Data Visualization for Understanding User Experience
Data visualization plays a crucial role in understanding user experience (UX) by providing a graphical representation of collected data. This technique allows for identifying patterns, trends, and relationships that may not be evident when analyzing the raw data. In this chapter, we will explore the importance of data visualization in UX and some effective techniques for representing information meaningfully.
Importance of Data Visualization in UX
Data visualization is fundamental in UX for several key reasons:
- Facilitates Understanding: Visual representations of data enable designers and UX professionals to quickly understand the information and extract meaningful insights.
- Identification of Patterns and Trends: Visualizations can reveal patterns and trends in the data that may be difficult to detect through manual analysis.
- Effective Communication: Visualizations help communicate findings and results to stakeholders and multidisciplinary teams clearly and concisely.
- Evidence-Based Decision Making: By providing a clear representation of data, visualizations allow for informed, evidence-based decision-making to improve UX.
Data Visualization Techniques in UX
There are various data visualization techniques that can be used to better understand user experience:
- Bar Charts and Pie Charts: These charts are useful for representing categorical data and comparing different categories to each other.
- Line Charts: Ideal for showing trends and changes in data over time, such as user behavior on a website or application.
- Heatmaps: These visualizations show the density and distribution of user interaction on an interface, highlighting areas of high activity.
- Flowcharts: Useful for representing the flow of users through a process, such as the navigation flow on a website or the sequence of actions in an application.
- Tree Diagrams: Allow visualizing the hierarchical structure of information and how users navigate through it.
Considerations When Visualizing Data in UX
When creating data visualizations to understand UX, it is important to consider some key considerations:
- Clarity and Simplicity: Visualizations should be clear and simple to facilitate quick understanding of the information.
- Relevance: Visualizations should be relevant to specific UX objectives and focus on the most important aspects for improving the user experience.
- Interactivity: The ability to interact with visualizations, such as zooming, filtering data, or viewing additional details, can significantly enhance their utility.
- Accessibility: It is important to ensure that visualizations are accessible to all users, including those with visual impairments.
In summary, data visualization is a powerful tool for understanding user experience by providing a clear and meaningful representation of collected information. By using effective data visualization techniques and considering the specific needs and objectives of UX, professionals can gain valuable insights to significantly improve the user experience.
Making Informed Decisions through Data
In today's technology-driven world, making informed decisions is essential for success in any field, and user experience (UX) design is no exception. Data collection and analysis are critical components for understanding user needs, preferences, and behaviors. In this chapter, we will explore how to use data to make informed decisions in UX design and how this practice can significantly improve the quality of the experiences offered.
Importance of Making Informed Decisions in UX
Making informed decisions in UX involves using data to support and guide design decisions. Some key reasons why this is crucial include:
- Precision in Design: Data provides precise information about user needs and behaviors, enabling designers to make more accurate and informed decisions.
- Risk Reduction: By basing decisions on real data rather than assumptions or intuition, the risk of developing products or services that do not meet user expectations is reduced.
- Idea Validation: Data can help validate design ideas and concepts before investing significant resources in their implementation, saving time and money.
- Continuous Improvement: Data collection and analysis allow for identifying areas for improvement in an existing experience, facilitating iteration and constant design evolution.
Data-Driven Decision-Making Process in UX
The process of making data-driven decisions in UX typically follows these steps:
- Goal Identification: Clearly define the design goals and the metrics that will be used to measure success.
- Data Collection: Use a variety of methods to collect relevant data, which may include surveys, interviews, user log analysis, and usability testing.
- Data Analysis: Examine and analyze the collected data to identify patterns, trends, and improvement opportunities.
- Insight Generation: Extract meaningful insights from the data to better understand user needs and behaviors.
- Decision-Making: Use the obtained insights to make informed decisions about UX design, prioritizing actions that have the greatest impact on the user experience.
Challenges in Data-Driven Decision-Making
While data-driven decision-making offers numerous benefits, it also presents some challenges, including:
- Data Quality: Ensuring the quality and integrity of the collected data is essential to ensure the accuracy of the insights obtained.
- Correct Interpretation: Data can be complex and difficult to interpret correctly, so it is important to have expertise in data analysis to extract meaningful insights.
- Balancing Quantitative and Qualitative: Finding a balance between quantitative and qualitative analysis is important to gain a comprehensive understanding of the user experience.
Benefits of Data-Driven Decision-Making in UX
Some of the key benefits of adopting a data-driven decision-making approach in UX include:
- Improved User Experience: By better understanding user needs and behaviors, it is possible to design more intuitive and satisfying experiences.
- Increased Conversion: Data-driven optimization can lead to an increase in conversions and user retention by eliminating friction points and improving user flow.
- Cost Reduction: Identifying areas for improvement through data analysis can help reduce costs associated with product and service development and maintenance.
In summary, making informed decisions in UX is essential for designing meaningful and effective experiences for users. By leveraging data to better understand user needs and behaviors, designers can create products and services that more effectively meet market demands and generate positive business outcomes.
Best Practices for Data-Driven UX
In user experience (UX) design, adopting a data-driven mindset is crucial for creating products and services that effectively meet user needs and expectations. Below, we'll explore some best practices to ensure that the design process is effectively backed by data.
Define Clear and Measurable Goals
Before embarking on any design project, it's crucial to establish clear and measurable goals that can guide the process. These goals should be specific, achievable, and aligned with user needs and business objectives. Additionally, they should be quantifiable so that they can be measured and the design's success evaluated.
Collect Relevant Data
Once the goals have been established, the next step is to collect relevant data that provides useful information about the user and their behavior. This may include demographic data, user preferences, product performance metrics, and user feedback. It's important to use a variety of data collection methods, such as surveys, interviews, usability testing, and user log analysis, to gain a comprehensive understanding of the user and their experience.
Effectively Analyze Data
Data analysis is a crucial part of the data-driven design process. Designers should be able to analyze and understand the collected data to extract meaningful insights that can inform the design. This involves using data analysis tools and techniques, as well as the ability to identify patterns, trends, and areas for improvement in the data.
Iterate and Continuously Improve
Data-driven UX is an iterative process where the design is continuously improved based on data and user feedback. Designers should be willing to make adjustments and improvements to the design based on data findings, with the goal of constantly optimizing the user experience. This may involve conducting A/B testing, iterating designs based on user feedback, and implementing changes based on performance metrics.
Create a Data-Driven Culture
Last but not least, it's important to create an organizational culture that values and prioritizes the use of data in the design process. This involves fostering collaboration between teams, promoting transparency in data usage, and encouraging all team members to use data to support their design decisions. By doing so, you can ensure that data-driven UX becomes an integral part of the company culture.
Future of Data-Driven UX
The evolution of user experience (UX) towards a more data-driven approach is undeniable and promises to further transform the way we design and develop digital products and services. In this chapter, we will explore some future trends and developments that will shape the landscape of data-driven UX in the coming years.
Artificial Intelligence and Machine Learning in UX
One of the most significant trends in the future of data-driven UX is the growing role of artificial intelligence (AI) and machine learning (ML). These technologies enable the quick and efficient analysis of large volumes of data, identifying patterns and trends, and predicting user behavior with greater accuracy. In the future, we will see increased use of AI and machine learning to personalize user experiences, automate design processes, and improve data-driven decision-making.
Conversational User Interface and Virtual Assistants
Another area that is gaining momentum is conversational user interface and virtual assistants. These technologies allow users to interact with digital systems more naturally, through speech or text, providing a more intuitive and personalized experience. In the future, we will see a rise in the development of data-driven virtual assistants that can understand and respond to user needs more effectively, providing contextualized recommendations and responses.
Immersive Experiences and Augmented Reality
Augmented reality (AR) and virtual reality (VR) are transforming how we interact with the digital world, and this will also impact data-driven UX. These technologies offer more immersive and engaging experiences, providing new opportunities to collect data on user behavior and preferences. In the future, we will see an increase in the development of AR and VR-based user experiences that leverage data to deliver highly personalized and contextualized experiences.
Ethics and Data Privacy
As data-driven UX continues to evolve, there is also a need to address ethical and data privacy concerns. It is crucial to ensure that data collection and usage are done ethically and transparently, protecting user privacy and security. In the future, we are likely to see a greater focus on data ethics and the implementation of robust privacy practices to ensure user trust in digital experiences.
Multidisciplinary Collaboration
Finally, the future of data-driven UX will require closer collaboration across different disciplines, including designers, data scientists, engineers, and ethics and privacy experts. This multidisciplinary collaboration will be essential to address complex challenges and leverage the full potential of data to significantly enhance user experience.