D Best Practices for Conducting A/B Tests in UX Design
Por Redacción Aguayo
This methodology not only allows us to make data-driven decisions but also eliminates guesswork, clearly showing us what works best for our users. However, running effective A/B tests isn’t just about launching two versions of something and seeing which one wins. It’s a meticulous, nuanced process that can make the difference between a marginal improvement and a quantum leap in conversion rates. 🚀
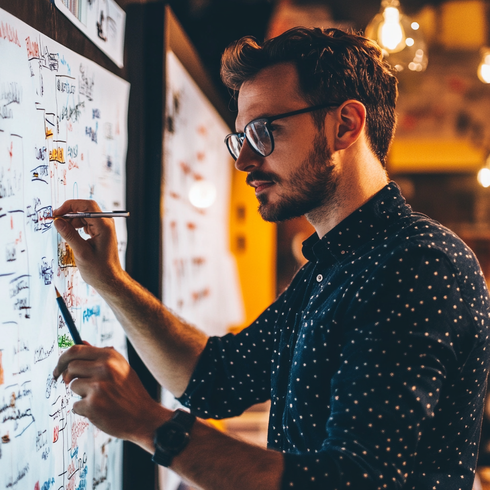
What Is an A/B Test and Why Is It Essential in UX?
An A/B test, also known as a split test, is a controlled experiment that compares two versions of a specific element of a digital product to determine which one performs better based on a defined metric. This element can be as simple as the color of a button or as complex as the design of a homepage, the structure of a form, a call-to-action (CTA), headline copy, or even an entire navigation flow.
The logic behind A/B testing is simple yet powerful: the audience is randomly divided into two groups. One group sees the original version, known as the “control,” while the other group interacts with the “variant,” which contains a specific change. Then, the behavior of both groups is measured based on predefined metrics, such as click-through rates, conversions, time on page, bounce rates, among others.
It may seem like a simple process, but its value lies in objectivity. Instead of making decisions based on intuition, assumptions, or the famous "I think that," A/B testing lets the data speak for itself. It’s the user, through their real and unaltered behavior, who shows us which version works better. This eliminates cognitive bias and allows UX, design, and product teams to make decisions grounded in concrete evidence.
The Importance of A/B Testing in UX
In the world of user experience design, every little detail can have a significant impact. A minor change in button color, font size, or message wording can influence how users interact with a product. A/B testing helps identify which adjustments lead to meaningful improvements and which do not.
Why Is A/B Testing Essential?
- Enables Continuous Improvement: Instead of undertaking complete redesigns, which can be costly and risky, A/B testing allows for controlled iteration. You can make small changes, measure their impact, and implement them permanently only if they prove effective.
- Reduces Risk: Rather than betting on a big idea without knowing if it will work, you can first test it with a sample of users to evaluate its real impact before a large-scale rollout.
- Promotes a Data-Driven Culture: Decisions no longer rely on personal opinions or internal hierarchies. Data becomes the ultimate judge, fostering a more scientific approach to decision-making.
- Improves User Experience: By identifying which design or functionality enhances user interaction, A/B testing helps optimize usability, creating more satisfying experiences.
A/B tests go beyond optimizing conversion or click-through rates. They also help better understand users, identify behavior patterns, and uncover insights that might otherwise go unnoticed.
Considerations Before Starting an A/B Test
Before diving into an A/B test, it’s crucial to prepare properly. An improvised approach can lead to inconclusive results—or worse, decisions based on misinterpreted data. Here are some key considerations to ensure your test is robust and reliable:
- Define Clear Objectives:
Before any test, ask yourself: What do I want to achieve?- Increase click-through rates on a button?
- Improve user retention on a page?
- Reduce cart abandonment rates?
- Defining a specific goal helps focus the test and objectively measure its success.
- Formulate Well-Founded Hypotheses:
Every A/B test should start with a thoughtful hypothesis. This isn’t about guessing—it’s about basing your assumptions on previous data, user behavior analysis, or UX design principles.
A good hypothesis follows this structure:
“If [I make this change], then [I expect this result] because [reason for the change].”
Example:
“If I change the button text from ‘Submit’ to ‘Get My Discount,’ clicks will increase because the new text is more persuasive and relevant to the user.” - User Segmentation:
Not all users are the same. A change that works well for one group may not have the same effect on another. Consider segmenting your test by factors such as:- Device type
- Geographic location
- Stage in the conversion funnel
- This approach yields more precise, actionable results.
- Basic Statistical Knowledge:
While you don’t need to be a statistician, understanding basic concepts is important:- Statistical Significance: Determines whether the results are truly conclusive or could be due to chance.
- Sample Size: A group that’s too small can produce misleading results. You need a large enough sample to detect significant differences.
- Sample Consistency:
Ensure each user sees the same version throughout their experience. If a user sees version A on one visit and version B on another, it can skew the results.
Considering these factors from the start will enhance the quality of your test and increase confidence in the results.
Designing the Test: How to Structure an Effective A/B Experiment
The design of the experiment is one of the most critical stages in the A/B testing process. How you structure your test will determine the validity and reliability of the results. Poor design can lead to confusing outcomes or, worse, incorrect conclusions.
Key Elements for Structuring an Effective A/B Test
- Control and Variant:
In every A/B test, the current version of your product or element is known as the “control.” The version that incorporates the change you want to evaluate is called the “variant.”
To obtain accurate results, it’s recommended to change only one element at a time. This allows you to clearly identify which specific factor is influencing user behavior. - Consistency in User Experience:
It’s essential for each user to have a consistent experience throughout the test. If a user sees version A during one visit and version B on another, it can introduce bias into the analysis.
Most A/B testing platforms manage this automatically using cookies or user identifiers. - Appropriate Test Duration:
Determining how long an A/B test should run is crucial.- Ending too soon may result in unrepresentative outcomes.
- Running too long can be affected by external factors like seasonal changes, marketing campaigns, or global events.
- Consider the following to determine the ideal duration:
- Traffic volume: The more traffic you have, the less time you’ll need to achieve statistically significant results.
- User behavior variability: If user behavior fluctuates (e.g., weekdays vs. weekends), ensure the test covers these cycles.
- Defining Success Metrics:
What metric will determine if the variant is better than the control? Metrics can vary based on the test’s goal:- Conversion Rate: Ideal for forms, shopping carts, landing pages, etc.
- Click-Through Rate (CTR): Perfect for buttons, links, CTAs, or ads.
- Time on Page or Bounce Rate: Useful for evaluating content quality or page usability.
- Controlling External Variables:
While you can’t control everything, try to minimize the impact of external factors that might skew results, such as launching an A/B test during a major marketing campaign or an unusual traffic period.
When to Consider Multivariate Testing
While A/B tests focus on comparing two versions with a single change, multivariate tests are useful when you want to test multiple changes simultaneously.
However, these tests require much higher traffic volumes to yield reliable results, as the number of combinations increases exponentially with each added variable.
Common A/B Testing Mistakes (and How to Avoid Them)
Even experienced UX professionals can make mistakes when conducting A/B tests. These errors not only invalidate results but can lead to data-driven decisions that negatively impact user experience and business performance. Identifying these pitfalls is the first step to avoiding them.
1. Changing Multiple Variables at Once
The Issue:
Modifying several elements simultaneously (e.g., changing a button’s color, CTA text, and content layout) leads to “variable confusion.” If performance changes, you won’t know which modification caused the result.
How to Avoid It:
- Make Incremental Changes: Test one variable at a time to isolate its impact.
- Use Multivariate Tests if Needed: If you must evaluate multiple variables simultaneously, use a multivariate test—just ensure you have enough traffic for statistical significance.
2. Stopping the Test Too Early
The Issue:
Impatience is the enemy of A/B testing. It’s tempting to end a test as soon as one variant seems to be winning. However, early results are often volatile due to random fluctuations in user behavior.
How to Avoid It:
- Predefine Test Duration: Set a minimum test period based on sample size and expected traffic (usually at least two full user behavior cycles, like complete weeks).
- Wait for Statistical Significance: Don’t make decisions until results are statistically significant, typically with a p-value less than 0.05. Also, consider effect size and consistency over time.
3. Ignoring Context
The Issue:
A variant’s performance can be influenced by external factors beyond design or functionality. Seasonal trends, special events, ongoing marketing campaigns, or device types can skew A/B test results.
How to Avoid It:
- Evaluate the Experiment’s Environment: Consider if external factors (like an ad campaign or global event) are affecting results.
- Segment Results: Analyze data by segments (device type, geographic location, acquisition channel) to identify specific patterns and avoid overgeneralizations.
- Run Tests at Different Times: If possible, conduct tests in different timeframes to validate whether results remain consistent over time.
Analyzing Results: Beyond the Numbers
Once an A/B test concludes, the most critical phase begins: data analysis. Many make the mistake of focusing solely on which variant “won” without digging deeper into the why. Good analysis goes beyond interpreting percentages or charts; it’s about uncovering meaningful insights that can inform future design decisions.
Statistical Significance
Statistical significance indicates the likelihood that the observed results are not due to chance. A p-value less than 0.05 is often the standard, meaning there’s less than a 5% probability that the results are random. However, relying solely on the p-value is a limited approach.
Key Aspects to Consider:
- Effect Size:
A result can be statistically significant but have minimal practical impact. Analyze whether the difference between variants is substantial enough to justify implementation. - Consistency of Results:
Check if the behavior remains stable over time and across different user segments. Inconsistent results may indicate external factors influencing the data.
Impact on the Overall User Experience
Sometimes, a variant may improve a specific metric, like click-through rate, but have negative side effects on other areas, such as user satisfaction or retention.
For example, an aggressive pop-up might boost conversions in the short term but cause frustration, reducing long-term user loyalty.
How to Evaluate the Overall Impact:
- Measure Multiple KPIs:
Don’t limit yourself to a single success metric. Assess the variant’s effect on different aspects of the user experience to get a comprehensive view. - Analyze User Behavior:
Dive into qualitative analysis. How are users interacting with the new variant? Are there patterns of abandonment or confusion?
Continuous Learning
A test that doesn’t yield significant results or where the variant doesn’t outperform the control is not a failure. On the contrary, it provides valuable insights into what doesn’t work—which can be as important as knowing what does.
Every A/B test is an opportunity to learn, iterate, and improve.
How to Foster Continuous Learning:
- Document Every Test:
Record the hypothesis, methodology, results, and key learnings. This creates a valuable knowledge base that helps prevent repeated mistakes and builds cumulative expertise. - Analyze Beyond the “Winners”:
Even if a test doesn’t show significant improvement, review the data for patterns, unexpected behaviors, or insights that could inspire future tests. - Iterate Based on Results:
Use learnings from each test as the foundation for new experiments. Optimization is a continuous process, not a one-time event.
Best Practices for Successful A/B Testing
After years of evolution in the UX field, several best practices have emerged to maximize the impact of A/B testing:
- Constant Iteration:
A/B testing isn’t a one-off event. The best companies run continuous tests to optimize every aspect of the user experience. - Test Prioritization:
Not all tests have the same impact. Use frameworks like ICE (Impact, Confidence, Ease) to prioritize which tests to run first.
Rigorous Documentation:
Keep detailed records of every test: hypothesis, methodology, results, and conclusions. This not only facilitates internal learning but also prevents the repetition of past mistakes.
A/B Testing on Mobile Devices: Challenges and Opportunities
User behavior on mobile devices can differ significantly from desktop, presenting unique challenges and opportunities for A/B testing.
- Load Speed:
Changes that affect load times can have a greater impact on mobile users due to varying connection speeds. - Screen Size:
Elements that work well on large screens might not perform the same on mobile. Ensure that designs are responsive and optimized for smaller displays. - Touch Interaction:
Consider how users interact with touchscreens. Small or poorly placed buttons can negatively affect the mobile experience.
Ethics in A/B Testing: Not Everything Goes
While the goal of A/B testing is to improve metrics, it’s essential to maintain ethical standards. Manipulating users emotionally or inducing undesired behaviors can have long-term consequences for brand reputation.
Ethical Considerations:
- Transparency:
In some cases, informing users that they’re part of an experiment may be the most ethical choice. - Data Privacy:
Ensure compliance with data protection regulations like GDPR, especially when collecting personal information.
User Impact:
Evaluate whether changes could negatively affect the user experience, even if they improve short-term business metrics.
Conclusion:
A/B testing is much more than a simple experiment to see which variant gets more clicks. It’s a powerful tool for understanding user behavior, optimizing the digital experience, and making decisions based on real data. However, its effectiveness doesn’t lie solely in technical execution but in the strategic approach: defining clear objectives, formulating strong hypotheses, rigorously analyzing results, and—most importantly—learning from every test, regardless of the outcome.
The true value of A/B testing in UX lies in its ability to foster a culture of continuous improvement. Each iteration, each piece of data collected, brings us closer to designing experiences that are not only functional but also meaningful to users. In an ever-evolving digital environment, curiosity, experimentation, and a commitment to quality are the best allies of any experience designer. This is where A/B testing truly shines—helping us transform assumptions into certainties and good ideas into exceptional experiences.